1. 文本解码原理--以MindNLP为例
1.1 自回归语言模型
- 根据前文预测下一个单词
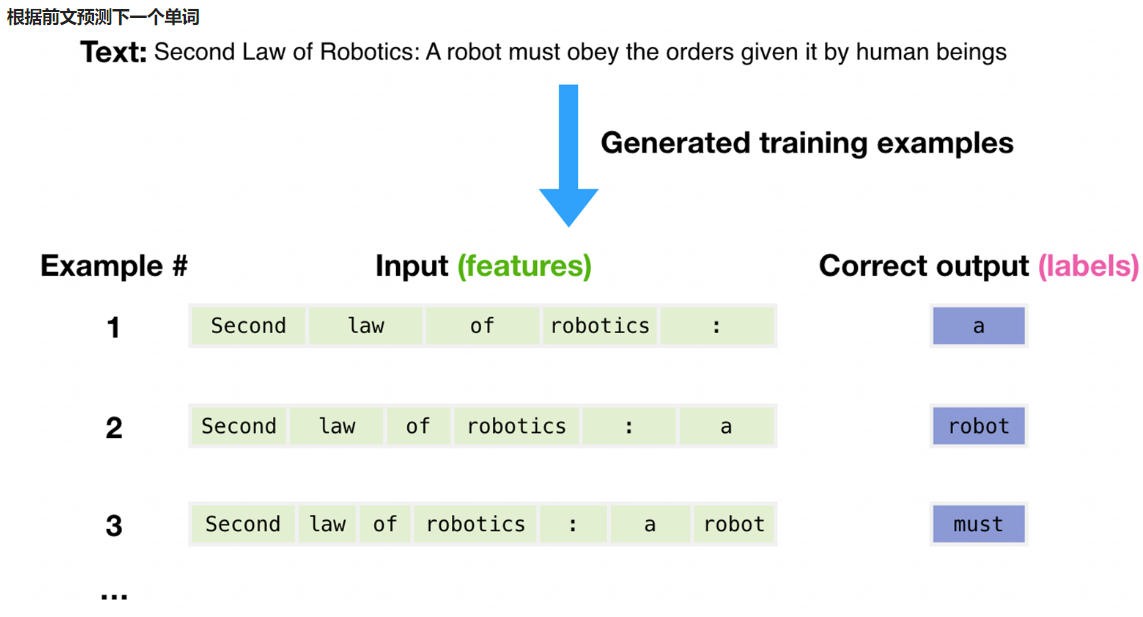
- 一个文本序列的概率分布可以分解为每个词基于其上文的条件概率的乘积

-
W 0 W_0 W0:初始上下文单词序列
-
t t t: 时间步
-
当生成EOS标签时,停止生成。
-
MindNLP/huggingface Transformers提供的文本生成方法
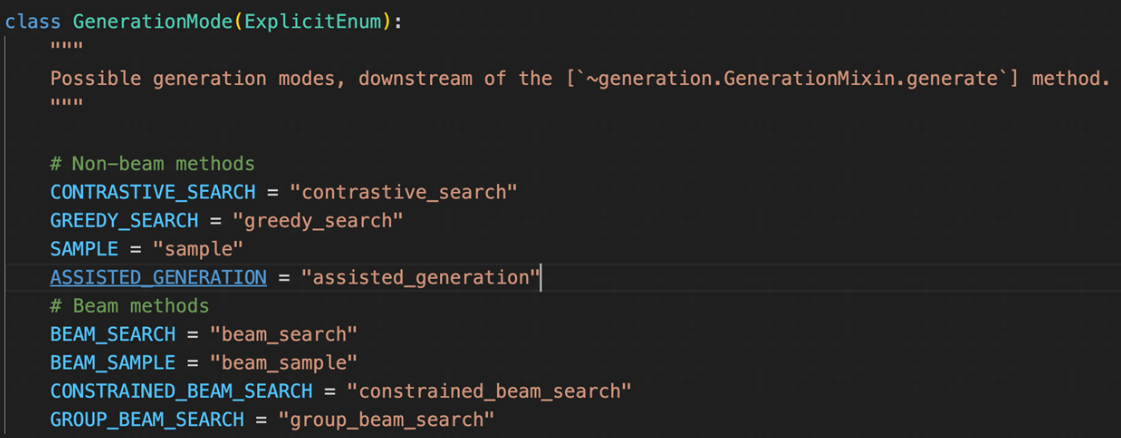
1.2 环境准备
!pip uninstall mindvision -y
!pip uninstall mindinsight -y
!pip install mindnlp
1.3 Greedy search(贪心搜索)
“Greedy search”(贪婪搜索)是一种在自然语言处理(NLP)和机器学习中的解码策略,特别是在文本生成任务中,如语言模型和机器翻译。在贪婪搜索中,每一步选择都是基于当前的最优可能性,而不考虑这个选择对未来的影响。
具体来说,在文本生成的上下文中,贪婪搜索会逐个token地生成文本,每次都选择概率最高的下一个token,而不考虑这个选择可能如何影响后续的token生成。这种方法简单且计算效率高,但可能会导致生成文本的质量不是最优的,因为它可能会错过那些当前概率稍低但长远来看会带来更好结果的token。
在每个时间步𝑡都简单地选择概率最高的词作为当前输出词:
w t w_t wt= a r g m a x w argmax_w argmaxw 𝑃( w w w| w w w 1 : t − 1 1:t-1 1:t−1)
按照贪心搜索输出序列(“The”,“nice”,“woman”) 的条件概率为:0.5 x 0.4 = 0.2
缺点: 错过了隐藏在低概率词后面的高概率词,如:dog=0.5, has=0.9
# 导入GPT2模型的分词器和模型
from mindnlp.transformers import GPT2Tokenizer, GPT2LMHeadModel# 初始化分词器,并加载预训练的权重,指定镜像源为modelscope
tokenizer = GPT2Tokenizer.from_pretrained("iiBcai/gpt2", mirror='modelscope')# 初始化模型,并加载预训练的权重,将EOS token设置为PAD token以避免警告,指定镜像源为modelscope
model = GPT2LMHeadModel.from_pretrained("iiBcai/gpt2", pad_token_id=tokenizer.eos_token_id, mirror='modelscope')# 对生成文本的条件上下文进行编码,得到input_ids
input_ids = tokenizer.encode('I enjoy walking with my cute dog', return_tensors='ms')# 使用贪婪搜索策略生成文本,直到输出长度(包括上下文长度)达到50
greedy_output = model.generate(input_ids, max_length=50)# 打印生成的文本
print("Output:\n" + 100 * '-')
print(tokenizer.decode(greedy_output[0], skip_special_tokens=True))
输出:
Building prefix dict from the default dictionary ...
Dumping model to file cache /tmp/jieba.cache
Loading model cost 1.036 seconds.
Prefix dict has been built successfully.
100%26.0/26.0 [00:00<00:00, 1.86kB/s]
100%0.99M/0.99M [00:00<00:00, 14.9MB/s]
100%446k/446k [00:00<00:00, 8.31MB/s]
100%1.29M/1.29M [00:00<00:00, 18.8MB/s]
100%665/665 [00:00<00:00, 66.8kB/s]
100%523M/523M [00:38<00:00, 11.4MB/s]
Output:
----------------------------------------------------------------------------------------------------
I enjoy walking with my cute dog, but I'm not sure if I'll ever be able to walk with my dog. I'm not sure if I'll ever be able to walk with my dog.I'm not sure if I'll
1.4 Beam search(束搜索)
Beam search(束搜索)是一种启发式搜索算法,常用于自然语言处理中的序列生成问题,如机器翻译和语音识别。与贪婪搜索不同,束搜索在每一步考虑多个可能的候选者,而不是只选择最优的一个。这样可以避免贪婪搜索可能会陷入的局部最优解问题。
束搜索的基本思想是保持一个大小为K的候选列表(称为“束”),在每一步都从这个列表中选出最有可能的K个候选者,然后基于这些候选者生成新的候选列表,如此迭代直到生成序列的结束。束的大小K称为束宽(beam width),是一个超参数,可以根据需要调整。
束搜索的优点是能够在一定程度上平衡探索(考虑多个候选者)和利用(选择当前最优候选者)之间的关系,从而生成质量更高的序列。但是,束搜索的计算复杂度比贪婪搜索要高,因为它需要维护一个候选列表,并且每一步都要对多个候选者进行评估。
在实际应用中,束搜索通常比贪婪搜索能产生更好的结果,因为它考虑了序列生成的全局性,而不是仅仅基于当前步骤的最优选择。然而,束搜索也可能错过最优解,因为它在某些情况下可能会丢弃一些最终可能成为最优解的候选者。因此,选择合适的束宽和搜索策略对于获得最佳结果至关重要。
Beam search通过在每个时间步保留最可能的 num_beams 个词,并从中最终选择出概率最高的序列来降低丢失潜在的高概率序列的风险。如图以 num_beams=2 为例:
(“The”,“dog”,“has”) : 0.4 * 0.9 = 0.36
(“The”,“nice”,“woman”) : 0.5 * 0.4 = 0.20
优点:一定程度保留最优路径
缺点:1. 无法解决重复问题;2. 开放域生成效果差
# 导入GPT2模型的分词器和模型
from mindnlp.transformers import GPT2Tokenizer, GPT2LMHeadModel# 初始化分词器,并加载预训练的权重,指定镜像源为modelscope
tokenizer = GPT2Tokenizer.from_pretrained("iiBcai/gpt2", mirror='modelscope')# 初始化模型,并加载预训练的权重,将EOS token设置为PAD token以避免警告,指定镜像源为modelscope
model = GPT2LMHeadModel.from_pretrained("iiBcai/gpt2", pad_token_id=tokenizer.eos_token_id, mirror='modelscope')# 对生成文本的条件上下文进行编码,得到input_ids
input_ids = tokenizer.encode('I enjoy walking with my cute dog', return_tensors='ms')# 使用束搜索和早停策略生成文本,设置束宽为5,最大长度为50
beam_output = model.generate(input_ids, max_length=50, num_beams=5, early_stopping=True
)# 打印生成的文本
print("Output:\n" + 100 * '-')
print(tokenizer.decode(beam_output[0], skip_special_tokens=True))
print(100 * '-')# 设置不重复n-gram的大小为2,以避免生成重复的文本
beam_output = model.generate(input_ids, max_length=50, num_beams=5, no_repeat_ngram_size=2, early_stopping=True
)# 打印生成的文本
print("Beam search with ngram, Output:\n" + 100 * '-')
print(tokenizer.decode(beam_output[0], skip_special_tokens=True))
print(100 * '-')# 设置返回多个序列的数量大于1
beam_outputs = model.generate(input_ids, max_length=50, num_beams=5, no_repeat_ngram_size=2, num_return_sequences=5, early_stopping=True
)# 打印所有生成的序列
print("return_num_sequences, Output:\n" + 100 * '-')
for i, beam_output in enumerate(beam_outputs):print("{}: {}".format(i, tokenizer.decode(beam_output, skip_special_tokens=True)))
print(100 * '-')
输出:
Output:
----------------------------------------------------------------------------------------------------
I enjoy walking with my cute dog, but I don't think I'll ever be able to walk with her again.""I don't think I'll ever be able to walk with her again.""I don't think I
----------------------------------------------------------------------------------------------------
Beam search with ngram, Output:
----------------------------------------------------------------------------------------------------
I enjoy walking with my cute dog, but I don't think I'll ever be able to walk with her again.""I'm not sure what to say to that," she said. "I mean, it's not like I'm
----------------------------------------------------------------------------------------------------
return_num_sequences, Output:
----------------------------------------------------------------------------------------------------
0: I enjoy walking with my cute dog, but I don't think I'll ever be able to walk with her again.""I'm not sure what to say to that," she said. "I mean, it's not like I'm
1: I enjoy walking with my cute dog, but I don't think I'll ever be able to walk with her again.""I'm not sure what to say to that," she said. "I mean, it's not like she's
2: I enjoy walking with my cute dog, but I don't think I'll ever be able to walk with her again.""I'm not sure what to say to that," she said. "I mean, it's not like we're
3: I enjoy walking with my cute dog, but I don't think I'll ever be able to walk with her again.""I'm not sure what to say to that," she said. "I mean, it's not like I've
4: I enjoy walking with my cute dog, but I don't think I'll ever be able to walk with her again.""I'm not sure what to say to that," she said. "I mean, it's not like I can
可以看到,使用束搜索后,回复多样性变差了,重复性变高
通过n-gram 惩罚: 将出现过的候选词的概率设置为 0 和设置no_repeat_ngram_size=2 ,任意 2-gram 不会出现两次。可以适当改善这个问题。Notice: 实际文本生成需要重复出现
1.5 Sample(样本搜索)
根据当前条件概率分布随机选择输出词 w t w_t wt
优点:文本生成多样性高
缺点:生成文本不连续
# 导入MindSpore库,用于设置随机种子
import mindspore# 导入GPT2模型的分词器和模型
from mindnlp.transformers import GPT2Tokenizer, GPT2LMHeadModel# 初始化分词器,并加载预训练的权重,指定镜像源为modelscope
tokenizer = GPT2Tokenizer.from_pretrained("iiBcai/gpt2", mirror='modelscope')# 初始化模型,并加载预训练的权重,将EOS token设置为PAD token以避免警告,指定镜像源为modelscope
model = GPT2LMHeadModel.from_pretrained("iiBcai/gpt2", pad_token_id=tokenizer.eos_token_id, mirror='modelscope')# 对生成文本的条件上下文进行编码,得到input_ids
input_ids = tokenizer.encode('I enjoy walking with my cute dog', return_tensors='ms')# 设置随机种子,以获得可重复的结果
mindspore.set_seed(0)# 激活采样策略,并关闭top_k采样,设置最大长度为50
sample_output = model.generate(input_ids, do_sample=True, max_length=50, top_k=0
)# 打印生成的文本
print("Output:\n" + 100 * '-')
print(tokenizer.decode(sample_output[0], skip_special_tokens=True))
输出:
Output:
----------------------------------------------------------------------------------------------------
I enjoy walking with my cute dog Neddy as much as I'd like. Keep up the good work Neddy!"I realized what Neddy meant when he first launched the website. "Thank you so much for joining."I
1.6 Temperature(温度)
降低softmax 的temperature使 P( w w w∣ w w w 1 : t − 1 1:t−1 1:t−1)分布更陡峭
增加高概率单词的似然并降低低概率单词的似然
# 导入MindSpore库,用于设置随机种子
import mindspore# 导入GPT2模型的分词器和模型
from mindnlp.transformers import GPT2Tokenizer, GPT2LMHeadModel# 初始化分词器,并加载预训练的权重,指定镜像源为modelscope
tokenizer = GPT2Tokenizer.from_pretrained("iiBcai/gpt2", mirror='modelscope')# 初始化模型,并加载预训练的权重,将EOS token设置为PAD token以避免警告,指定镜像源为modelscope
model = GPT2LMHeadModel.from_pretrained("iiBcai/gpt2", pad_token_id=tokenizer.eos_token_id, mirror='modelscope')# 对生成文本的条件上下文进行编码,得到input_ids
input_ids = tokenizer.encode('I enjoy walking with my cute dog', return_tensors='ms')# 设置随机种子,以获得可重复的结果
mindspore.set_seed(1234)# 激活采样策略,并关闭top_k采样,设置最大长度为50,设置温度系数为0.7
sample_output = model.generate(input_ids, do_sample=True, max_length=50, top_k=0,temperature=0.7
)# 打印生成的文本
print("Output:\n" + 100 * '-')
print(tokenizer.decode(sample_output[0], skip_special_tokens=True))
输出:
Output:
----------------------------------------------------------------------------------------------------
I enjoy walking with my cute dog and have never had a problem with her until now.A large dog named Chucky managed to get a few long stretches of grass on her back and ran around with it for about 5 minutes, ran around
1.7 TopK sample(TopK采样)
Top-K采样是一种在自然语言处理中常用的采样策略,尤其是在文本生成任务中。它的基本思想是在生成每个token时,从模型预测的下一个token的概率分布中选取概率最高的K个token,然后根据这K个token的概率分布进行采样。
选出概率最大的 K 个词,重新归一化,最后在归一化后的 K 个词中采样
将采样池限制为固定大小 K :
- 在分布比较尖锐的时候产生胡言乱语
- 在分布比较平坦的时候限制模型的创造力
# 导入MindSpore库,用于设置随机种子
import mindspore# 导入GPT2模型的分词器和模型
from mindnlp.transformers import GPT2Tokenizer, GPT2LMHeadModel# 初始化分词器,并加载预训练的权重,指定镜像源为modelscope
tokenizer = GPT2Tokenizer.from_pretrained("iiBcai/gpt2", mirror='modelscope')# 初始化模型,并加载预训练的权重,将EOS token设置为PAD token以避免警告,指定镜像源为modelscope
model = GPT2LMHeadModel.from_pretrained("iiBcai/gpt2", pad_token_id=tokenizer.eos_token_id, mirror='modelscope')# 对生成文本的条件上下文进行编码,得到input_ids
input_ids = tokenizer.encode('I enjoy walking with my cute dog', return_tensors='ms')# 设置随机种子,以获得可重复的结果
mindspore.set_seed(0)# 激活采样策略,并关闭top_k采样,设置最大长度为50,设置top_k为50
sample_output = model.generate(input_ids, do_sample=True, max_length=50, top_k=50
)# 打印生成的文本
print("Output:\n" + 100 * '-')
print(tokenizer.decode(sample_output[0], skip_special_tokens=True))
输出:
Output:
----------------------------------------------------------------------------------------------------
I enjoy walking with my cute dog.She's always up for some action, so I have seen her do some stuff with it.Then there's the two of us.The two of us I'm talking about were
1.8 Top-P sample(Top-P采样)
Top-P(Nucleus)采样是一种在自然语言处理中用于文本生成的采样策略。与Top-K采样不同,Top-P采样考虑的是概率分布的累积概率,而不是直接选择概率最高的K个token。
在Top-P采样中,模型会考虑概率分布中前P个token的累积概率,然后从这个概率累积的“核”中进行采样。具体来说,对于每个token,如果其概率小于等于前P个token的累积概率,那么它就会被包含在“核”中,模型会从这个“核”中采样下一个token。
Top-P采样允许模型在生成文本时考虑概率分布的大部分内容,同时仍然能够生成一些概率较低但可能有趣的token。这样可以增加生成文本的多样性,同时避免完全随机采样可能导致的噪声。
在累积概率超过概率 p 的最小单词集中进行采样,重新归一化
采样池可以根据下一个词的概率分布动态增加和减少
# 导入MindSpore库,用于设置随机种子
import mindspore# 导入GPT2模型的分词器和模型
from mindnlp.transformers import GPT2Tokenizer, GPT2LMHeadModel# 初始化分词器,并加载预训练的权重,指定镜像源为modelscope
tokenizer = GPT2Tokenizer.from_pretrained("iiBcai/gpt2", mirror='modelscope')# 初始化模型,并加载预训练的权重,将EOS token设置为PAD token以避免警告,指定镜像源为modelscope
model = GPT2LMHeadModel.from_pretrained("iiBcai/gpt2", pad_token_id=tokenizer.eos_token_id, mirror='modelscope')# 对生成文本的条件上下文进行编码,得到input_ids
input_ids = tokenizer.encode('I enjoy walking with my cute dog', return_tensors='ms')# 设置随机种子,以获得可重复的结果
mindspore.set_seed(0)# 激活采样策略,并关闭top_k采样,设置最大长度为50,设置top_p为0.92,设置top_k为0
sample_output = model.generate(input_ids, do_sample=True, max_length=50, top_p=0.92, top_k=0
)# 打印生成的文本
print("Output:\n" + 100 * '-')
print(tokenizer.decode(sample_output[0], skip_special_tokens=True))
输出:
Output:
----------------------------------------------------------------------------------------------------
I enjoy walking with my cute dog Neddy as much as I'd like. Keep up the good work Neddy!"I realized what Neddy meant when he first launched the website. "Thank you so much for joining."I
1.9 top_k_top_p(混合采样)
top_k_top_p是一种在自然语言处理中用于文本生成的混合采样策略,结合了Top-K采样和Top-P(Nucleus)采样。这种策略允许模型在生成文本时同时考虑概率分布中的高概率token和累积概率的“核”。
在Top-K采样中,模型会考虑概率分布中前K个token的累积概率,然后从这个概率累积的“核”中进行采样。而在Top-P采样中,模型会考虑概率分布中前P个token的累积概率,然后从这个概率累积的“核”中进行采样。
top_k_top_p采样策略结合了这两种策略的优点。具体来说,它首先从概率分布中选择K个最可能的token,然后从这些token中选择概率最高的P个token进行采样。这种方法既考虑了概率分布的主要部分,又保留了生成的多样性。
# 导入MindSpore库,用于设置随机种子
import mindspore# 导入GPT2模型的分词器和模型
from mindnlp.transformers import GPT2Tokenizer, GPT2LMHeadModel# 初始化分词器,并加载预训练的权重,指定镜像源为modelscope
tokenizer = GPT2Tokenizer.from_pretrained("iiBcai/gpt2", mirror='modelscope')# 初始化模型,并加载预训练的权重,将EOS token设置为PAD token以避免警告,指定镜像源为modelscope
model = GPT2LMHeadModel.from_pretrained("iiBcai/gpt2", pad_token_id=tokenizer.eos_token_id, mirror='modelscope')# 对生成文本的条件上下文进行编码,得到input_ids
input_ids = tokenizer.encode('I enjoy walking with my cute dog', return_tensors='ms')# 设置随机种子,以获得可重复的结果
mindspore.set_seed(0)# 激活采样策略,并设置top_k为5,top_p为0.95,设置最大长度为50,设置num_return_sequences为3
sample_outputs = model.generate(input_ids,do_sample=True,max_length=50,top_k=5,top_p=0.95,num_return_sequences=3
)# 打印生成的文本
print("Output:\n" + 100 * '-')
for i, sample_output in enumerate(sample_outputs):print("{}: {}".format(i, tokenizer.decode(sample_output, skip_special_tokens=True)))
print(100 * '-')
输出:
2. 小结
本文以MindNLP为例,介绍了大语言模型的解码原理。包括贪心搜索、束搜索、样本搜索、温度、TopK采样方法、Top-P采样方法、混合采样方法等对解码结果的影响。